Keynote Speakers
Two exciting keynotes will be given by Maria Gorlatova (Duke University) and Nikolas Kourtellis (Telefonica Research).
Intelligent Mobile Augmented Reality: Promise, Challenges, and Solutions
Maria Gorlatova
Duke University
Mobile augmented reality (AR), which integrates virtual objects with 3D real environments in real time, has been showing outstanding potential in many application areas including education, retail, and medicine. A key part of the so-called Metaverse that is being developed by multiple companies including Apple, Meta, Google, and Microsoft, AR is broadly expected to redefine how humans interact with the world around them. Yet current AR falls short of many of the expectations. This talk presents our vision for multi-device edge computing-supported architectures for next-generation intelligent AR. The talk describes shortcomings in modern AR’s semantic, spatial, and user context awareness capabilities, and describes how a combination of advances in edge computing and machine learning can address some of the key challenges. The talk showcases several next-generation AR applications, including AR in surgery and mental health.
Will it be Privacy or Utility? Can I have both (with Privacy-Preserving ML) please?
Nikolas Kourtellis
Telefonica Research
In this talk, I will dive into the realm of privacy-preserving machine learning (PPML) and cover recent advances that have the potential to unlock the power of user data for companies, while still preserving the privacy of the data owners. I will discuss major tradeoffs between privacy and machine learning utility of PPML and potential adversarial attacks that can be mounted on such modeling. In particular, I will talk about technology we have been building based on Federated Learning (FL), Differential Privacy (DP), Trusted Execution Environments (TEE), Edge Computing (EC) and detailed system design, engineering and experimentation. I will present the design, building and testing of the first Federated Learning as a Service platform (FLaaS) which supports on-device training between mobile apps, the first Privacy-Preserving FL solution (PPFL) based on TEEs, and the first Hierarchical FL solution with DP (HFL). I will elaborate on their ML modeling functionalities, performance and system overhead tradeoffs, and how thheir privacy-preserving design can mitigate such adversarial data modeling attacks without compromising model utility.
-
Keynote Speakers
Maria Gorlatova
Duke UniversityNikolas Kourtellis
Telefonica Research - Paper Submission Deadline:
April 8th - 11:59PM AOE
April 22nd - 11:59PM AOE (Final) - Workshop Event:
July 1st 2022
Call for Papers [PDF]
Committees
Keynote Talks
Attendee Information
Technical Program
Prior Workshops
Submissions
Important Dates
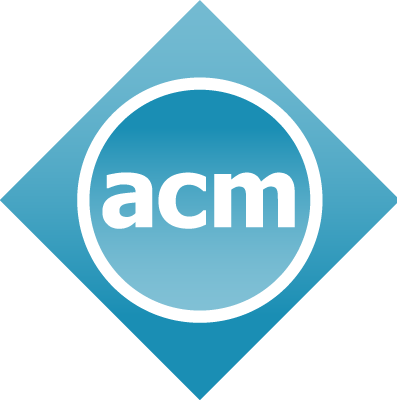
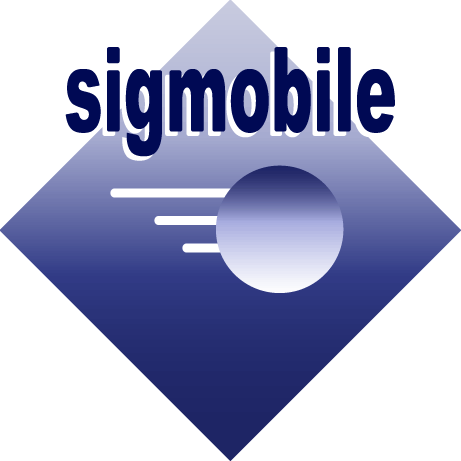